A look at how you should price a bet
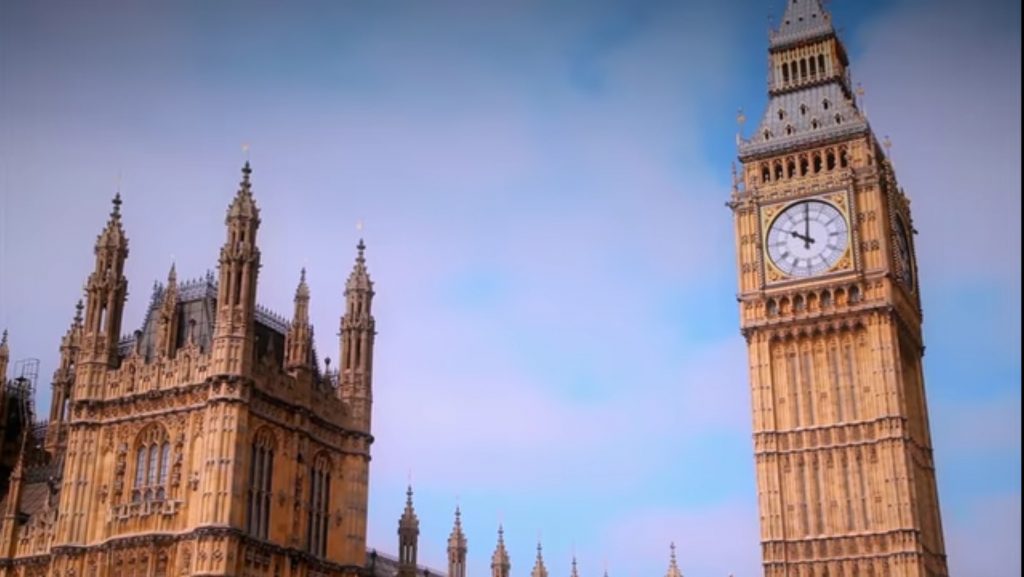
Part One – The bet
Earlier this year, several PBers, including OGH, bet on there being a Labour lead by the end of the year. (They won, because of the opinion poll covered here. Well done!).
More recently, Smarkets has established a market on the prospects of a Conservative lead by end of January (covered here).
But this bet (‘the bet’) is perhaps the most interesting out there from a theoretical point of view.
When it offers good value is partly a mathematical question, but luck and judgement are also important. I will set out the theory and practice in four threads:
- This part looks at the theory of the pricing
- The second part will present a simplified worked example
- The third part will examine historic evidence on the volatility of opinion polls, which is an important input variable and interesting in itself
- The fourth part will look at the possibility of serial autocorrelation in the change in Labour leads and its implications for the bet, and at the implications of there being more than one polling company.
Assumptions
For this article, I assume, for simplicity’s sake, that:
- there is no particular reason to expect a change in Labour’s lead one way or the other (in statistical jargon, the expected drift is zero)
- accordingly, the change in the lead, L, over time t, follows stochastic Brownian motion, i.e. the change between polls is distributed normally, mean 0, standard deviation ?, or, formally,
dL/dt = Z(0,?)
- there is only one series of polls, meaning we can ignore the possibility that some pollsters are likely to show higher, or lower, Labour leads than others conducted at the same time
- opinion polls are discrete events, not continuous. Unlike, say, the price of a liquid financial asset, which is traded more or less continuously, opinion polls happen every few days or weeks
Excel modelling as a shortcut
The mathematics for this bet is extremely difficult, but fortunately, for those with access to Excel, there is a quick way to find approximate probabilities: use the random number generator to model stochastic Brownian motion for a large number of scenarios (the NORM_INV and RAND() functions).
With enough runs (I recommend at least five thousand – all you need to do is copy and paste across and down the spreadsheet) random variations will even out and the results obtained will be robust to a tolerance of 1% or even 0.5% – this should be adequate for most punters given the uncertainty in the variables in this calculation.